In recent years, artificial intelligence (AI) has made significant strides, impacting various sectors, including healthcare, finance, and entertainment. One of the most promising areas where AI is making a substantial difference is education. By leveraging AI technologies, educational institutions and learners are experiencing a transformation in how education is delivered, accessed, and personalized. In this blog post, we’ll explore how AI is being integrated into education, its technical underpinnings, and the potential benefits and challenges associated with its implementation.
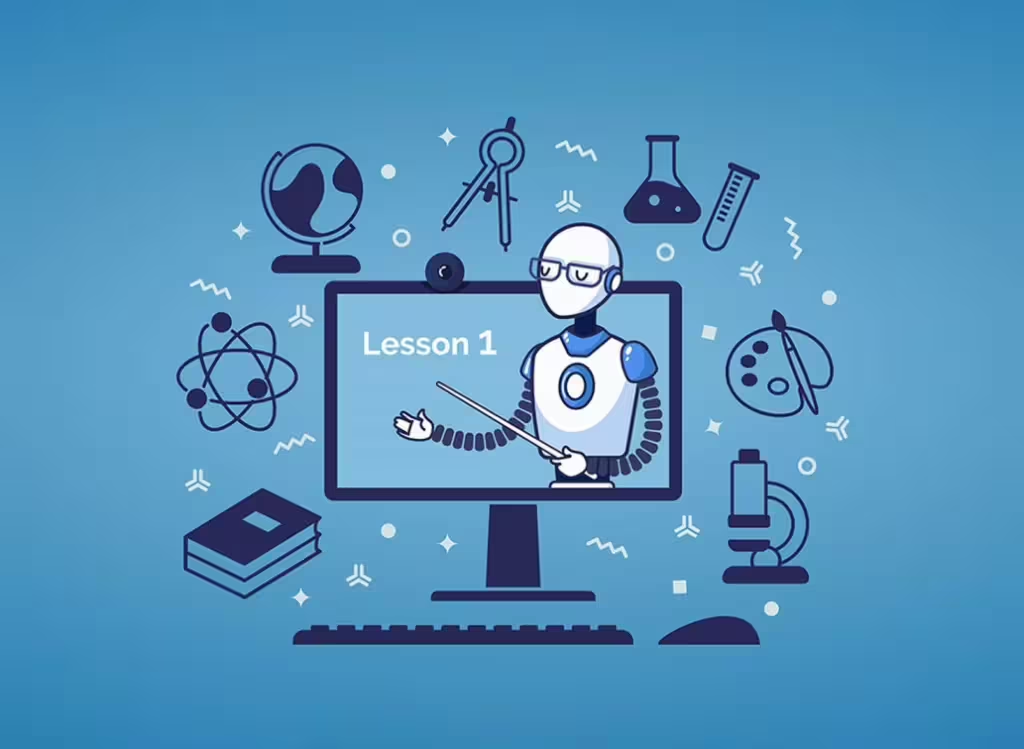
What is AI in Education?
AI in education refers to the use of artificial intelligence technologies to enhance and support teaching and learning processes. Technically, AI encompasses a range of techniques and algorithms that enable machines to perform tasks that typically require human intelligence. In the context of education, this includes:
- Natural Language Processing (NLP): Allows machines to understand and generate human language. In education, NLP can be used for automatic essay grading, language translation, and creating interactive chatbots.
- Machine Learning (ML): A subset of AI that involves training algorithms on data to make predictions or decisions without explicit programming. In education, ML algorithms can analyze student performance data to provide personalized learning recommendations.
- Adaptive Learning Systems: These systems use AI to tailor educational content and resources to meet the individual needs of students based on their learning styles and progress.
- Robotic Process Automation (RPA): In education, RPA can automate administrative tasks such as scheduling, grading, and handling routine inquiries, freeing up educators to focus on teaching.
How AI is Revolutionizing Education
- Personalized Learning: One of the most significant advantages of AI in education is its ability to create personalized learning experiences. Adaptive learning platforms use AI algorithms to analyze a student’s strengths, weaknesses, and learning preferences. Based on this analysis, these systems adjust the difficulty level of tasks, suggest relevant resources, and provide customized feedback. This tailored approach helps address individual learning gaps and improves overall educational outcomes.
- Intelligent Tutoring Systems: AI-powered tutoring systems simulate one-on-one instruction by providing immediate feedback and support. These systems can interact with students, answer questions, and offer explanations in real-time, mimicking the role of a human tutor. For example, platforms like Carnegie Learning’s MATHia use AI to guide students through math problems and offer personalized assistance.
- Automated Grading and Assessment: Grading assignments and exams can be a time-consuming task for educators. AI can streamline this process through automated grading systems that evaluate student work based on predefined criteria. These systems can assess multiple-choice questions, essays, and even coding assignments, providing timely and consistent feedback.
- Enhanced Engagement through Gamification: AI can also enhance student engagement by integrating gamification elements into educational content. AI algorithms can create adaptive learning games that adjust difficulty levels based on student performance, making learning more interactive and enjoyable.
- Language Translation and Accessibility: AI-driven language translation tools can break down language barriers in education. By providing real-time translation of educational materials and lectures, AI helps make learning more accessible to non-native speakers and students with diverse linguistic backgrounds.
Technical Underpinnings
- Data Collection and Analysis: AI systems in education rely heavily on data collection and analysis. Student performance data, interaction patterns, and feedback are collected and analyzed to inform AI algorithms. Machine learning models are trained on this data to recognize patterns and make predictions.
- Natural Language Processing: NLP techniques, such as sentiment analysis and language generation, are employed to understand and respond to student queries and provide feedback. For example, sentiment analysis can gauge the emotional tone of student responses to tailor feedback accordingly.
- Algorithm Development: AI systems use various algorithms, including neural networks and decision trees, to process educational data and make recommendations. These algorithms are developed and refined through training on large datasets to improve accuracy and effectiveness.
Benefits and Challenges
Benefits:
- Personalization: Tailors learning experiences to individual needs, improving student outcomes.
- Efficiency: Automates administrative tasks, saving time for educators.
- Accessibility: Provides language support and resources to diverse student populations.
- Engagement: Enhances learning through interactive and gamified content.
Challenges:
- Data Privacy: Ensuring the security and privacy of student data is crucial.
- Bias: AI systems can inadvertently perpetuate biases present in training data, leading to unfair outcomes.
- Cost: Implementing AI technologies can be expensive, potentially widening the gap between well-funded and underfunded institutions.
- Dependence on Technology: Over-reliance on AI may impact the development of critical thinking and problem-solving skills.
Conclusion
AI in education holds immense potential to transform the way we teach and learn. By leveraging advanced technologies such as natural language processing, machine learning, and adaptive learning systems, educators can offer more personalized, efficient, and engaging learning experiences. However, it is essential to address the challenges associated with AI implementation to ensure its benefits are realized equitably and ethically. As AI continues to evolve, its role in education will likely expand, offering new opportunities to enhance the learning journey for students around the world.
Leave a Reply