n the ever-evolving landscape of cybersecurity, the introduction of Artificial Intelligence (AI) and Machine Learning (ML) technologies has marked a significant paradigm shift. The sophistication and volume of cyber threats have surged, demanding more advanced and adaptive security solutions. AI and ML are no longer mere supplementary tools but integral components of next-generation cybersecurity strategies. This blog post delves into how AI and ML are transforming cybersecurity, enhancing threat detection, response, and overall defense mechanisms.
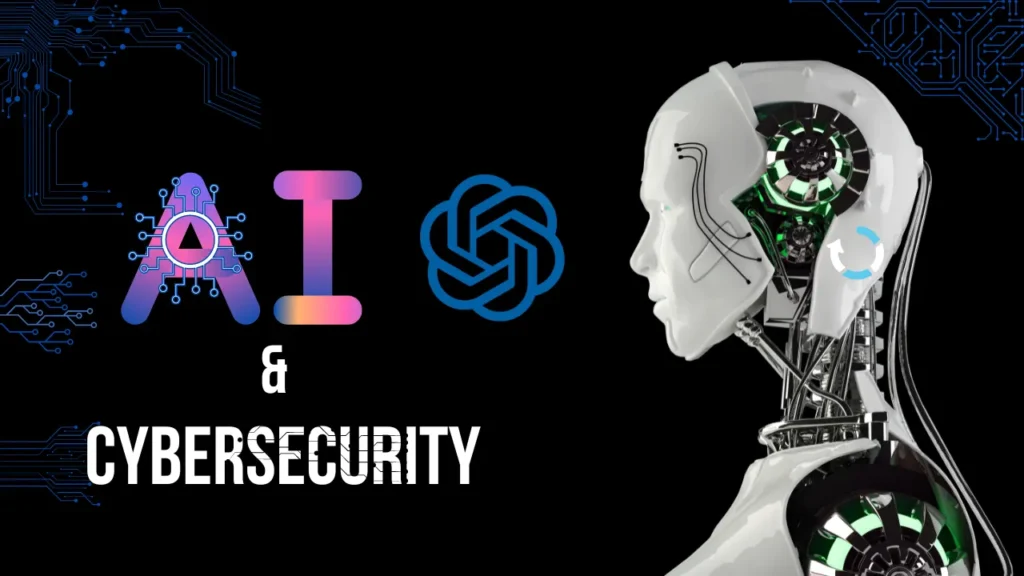
The Evolution of Cyber Threats
The complexity and scale of cyber threats have grown exponentially. Traditional security measures, such as signature-based detection and rule-based systems, struggle to keep pace with modern attack vectors. Cyber attackers now employ advanced techniques, including polymorphic malware, zero-day exploits, and sophisticated phishing schemes, making traditional defenses less effective. To counter these evolving threats, cybersecurity must leverage AI and ML to stay ahead of the curve.
AI and ML: A Game-Changer for Cybersecurity
AI and ML technologies provide a more dynamic approach to cybersecurity, characterized by adaptive learning and real-time threat analysis. Here’s how these technologies are revolutionizing the field:
1. Advanced Threat Detection
Behavioral Analysis: Traditional systems rely on known signatures to detect threats, which is inadequate for zero-day attacks and sophisticated malware. AI and ML enhance threat detection through behavioral analysis, monitoring deviations from established patterns of normal behavior. For example, ML algorithms can analyze network traffic and identify anomalies that deviate from typical user activity, flagging potential threats that conventional systems might miss.
Anomaly Detection: Machine learning models excel at identifying anomalies by learning from historical data. They can detect unusual patterns in real-time, such as atypical login times or unexpected data access, which could indicate a breach or insider threat. This proactive approach allows for the identification of novel threats that do not match existing signatures.
2. Predictive Analytics and Threat Intelligence
Predictive Threat Modeling: ML algorithms can analyze vast amounts of data to predict potential threats based on historical patterns and emerging trends. By identifying indicators of compromise (IoCs) and tactics, techniques, and procedures (TTPs) used by threat actors, predictive models help organizations anticipate and prepare for future attacks.
Threat Intelligence Integration: AI can aggregate and analyze threat intelligence from various sources, including dark web forums, security reports, and global threat databases. By correlating this data with internal network activity, AI enhances the accuracy of threat predictions and provides actionable insights for preemptive measures.
3. Automated Response and Mitigation
Incident Response Automation: AI-driven systems can automate response actions to detected threats, reducing the time from detection to mitigation. For example, when a security incident is detected, an AI system might automatically isolate the affected network segment, block malicious IP addresses, or deploy additional security measures to contain the threat.
Adaptive Security Policies: Machine learning models continuously learn from new threat data and adjust security policies accordingly. This dynamic adjustment ensures that security measures evolve in response to emerging threats, maintaining robust defenses without manual intervention.
4. Enhanced Phishing and Malware Protection
Phishing Detection: AI and ML algorithms are adept at analyzing email content, URLs, and user behavior to identify phishing attempts. Natural Language Processing (NLP) models can scrutinize email text for suspicious patterns, while machine learning models assess URL reputation and user interactions to filter out malicious content.
Malware Analysis: ML-based malware analysis tools can detect and classify new variants of malware by analyzing their behavior and characteristics. Unlike traditional signature-based methods, which rely on known malware signatures, ML models adapt to identify and mitigate previously unknown threats.
Challenges and Considerations
While AI and ML offer substantial benefits, their integration into cybersecurity also presents challenges:
Data Privacy: The deployment of AI in cybersecurity requires access to extensive data, which raises concerns about data privacy and compliance with regulations such as GDPR and CCPA. Organizations must ensure that AI systems are designed to handle data responsibly and securely.
Model Bias and Accuracy: Machine learning models are susceptible to biases in training data, which can impact their accuracy and effectiveness. Continuous monitoring and refinement of models are necessary to mitigate biases and maintain high performance.
Complexity and Resource Requirements: Implementing AI and ML solutions requires significant computational resources and expertise. Organizations must invest in the necessary infrastructure and skilled personnel to effectively deploy and manage these technologies.
The Road Ahead: AI and ML in Cybersecurity
The future of cybersecurity is increasingly intertwined with AI and ML. As cyber threats continue to evolve, these technologies will play a crucial role in developing more resilient and adaptive security systems. Advancements in AI and ML will enable more sophisticated threat detection, faster response times, and greater predictive capabilities, enhancing overall cybersecurity posture.
Organizations that leverage AI and ML effectively will be better equipped to anticipate, detect, and respond to emerging threats, staying ahead of adversaries in the ongoing battle for digital security. Embracing these technologies not only strengthens defenses but also positions organizations for success in a rapidly changing cybersecurity landscape.
As AI and ML continue to advance, their integration into cybersecurity strategies will be essential for achieving next-generation protection and maintaining robust defenses against ever-evolving cyber threats.
Leave a Reply